ALifeE (Artificial Life Environment) is a cognitive architecture, including virtual sensors and perception, learning methods to approximate behavioral and cognitive models for Autonomous Virtual Agents (AVA), developed by Toni Conde in the Virtual Reality Laboratory (VRLab), (founder and director - Professor Daniel Thalmann), at the Swiss Federal Institute of Technology (Ecole Polytechnique Fédérale de Lausanne - EPFL) in Lausanne, Switzerland.
E-mail: Этот адрес электронной почты защищён от спам-ботов. У вас должен быть включен JavaScript для просмотра. | Toni Conde's Profile | T. Conde‘s profile on scholar.google.ru
Когнитивная архитектура ALifeE разрабатывалась под руководством Тони Конде (Toni Conde) в Лаборатории виртуальной реальности (VRLab), основателем и директором которой был профессор Даниэл Талманн (Daniel Thalmann), которая находится в Швейцарской высшей политехнической школе Лозанны (Ecole Polytechnique Fédérale de Lausanne - EPFL).
С января 2011 года деятельность лаборатории приостановлена в связи с отставкой профессора Даниэла Талманна, дальнейшая деятельность ведется в рамках исследовательской группы Immersive Interaction research Group (IIG).
Д. Талманн продолжает свои исследования в Наньянском технологическом университете в Сингапуре (смотри ниже).
Тони Конде продолжает работу в коммерческом секторе (смотри его профиль).
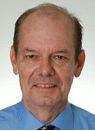
Email: Этот адрес электронной почты защищён от спам-ботов. У вас должен быть включен JavaScript для просмотра.
Homepage and CV at EPFL (Daniel_Thalmann wiki)
He is currently here:
Prof. Daniel Thalmann
Institute for Media Innovation (IMI)
Nanyang Technological University (NTU) in Singapore
Email: Этот адрес электронной почты защищён от спам-ботов. У вас должен быть включен JavaScript для просмотра. | D.Thalmann's Profile | D. Thalmann‘s profile on scholar.google.ru
Общие сведения
Диссертация Тони Конде (выдержки) Thèse_Conde_Toni - Full text (pdf, 2 MiB)
There are two primary approaches to behavioural animation of an Autonomous Virtual Agent (AVA).The first one, or behavioural model, defines how AVA reacts to the current state of its environment.
In the second one, or cognitive model, this AVA uses a thought process allowing it to deliberate over its possible actions.Despite the success of these approaches in several domains, there are two notable limitations which we address in this thesis.
First, cognitive models are traditionally very slow to execute, as a tree search, in the form of mapping: states ¨ actions, must be performed.
On the one hand, an AVA can only make sub-optimal decisions and, on the other hand, the number of AVAs that can be used simultaneously in real-time is limited.
These constraints restrict their applications to a small set of candidate actions. Second, cognitive and behavioural models can act unexpectedly, producing undesirable behaviour in certain regions of the state space. This is because it may be impossible to exhaustively test them for the entire state space, especially if the state space is continuous. This can be worrisome for end-user applications involving AVAs, such as training simulators for cars and aeronautics.
Our contributions include the design of novel learning methods for approximating behavioural and cognitive models. They address the problem of input selection helped by a novel architecture ALifeE including virtual sensors and perception, regardless of the machine learning technique utilized. The input dimensionality must be kept as small as possible, this is due to the "curse of dimensionality", well known in machine learning. Thus, ALifeE simplifies and speeds up the process for the designer.
Contract/grant sponsor: Swiss National Science Foundation.
Selected Publications
1. List of publications at EPFL
2. Toni Conde, Daniel Thalmann. An Artificial Life Environment for Autonomous Virtual Agents
http://infoscience.epfl.ch/record/98985/files/Conde_Thalmann_cavw_04.pdf
3. Toni Conde, Daniel Thalmann. Learnable Behavioural Model for Autonomous Virtual Agents: Low-Level Learning
http://infoscience.epfl.ch/record/99036/files/Conde_Thalmann_AAMAS_06.pdf